Automated Segmentation and Quantification of the Right Ventricle in 2-D Echocardiography
Chernyshov A, Grue JF, Nyberg J, Grenne B, Dalen H, Aase SA, Østvik A, Lovstakken L
In the work described we developed deep learning methods for automated segmentation and extraction of key clinical paremeters from the right ventricle. In particular, we explored a keypoint detection approach to segmentation that guards against erratic behavior often displayed by current segmentation models.
We used a data set of echo images focused on the right ventricle from 250 participants to train and evaluate several deep learning models. We proposed a compact architecture (U-Net KP) employing the keypoint approach, designed to balance high speed with accuracy and robustness. All featured models achieved segmentation accuracy close to the inter-observer variability. When computing the metrics of right ventricular systolic function from contour predictions of U-Net KP, we obtained the bias and 95% limits of agreement of 0.8 ± 10.8% for the right ventricular fractional area change measurements, –0.04 ± 0.54 cm for the tricuspid annular plane systolic excursion measurements and 0.2 ± 6.6% for the right ventricular free wall strain measurements. These results were also comparable to the semi-automatically derived inter-observer discrepancies of 0.4 ± 11.8%, –0.37 ± 0.58 cm and –1.0 ± 7.7% for the aforementioned metrics respectively.
In conclusion, given the appropriate data, automated segmentation and quantification of the right ventricle in 2-D echocardiography proved feasible with existing methods. Further, keypoint detection architectures may offer higher robustness and information density for the same computational cost.
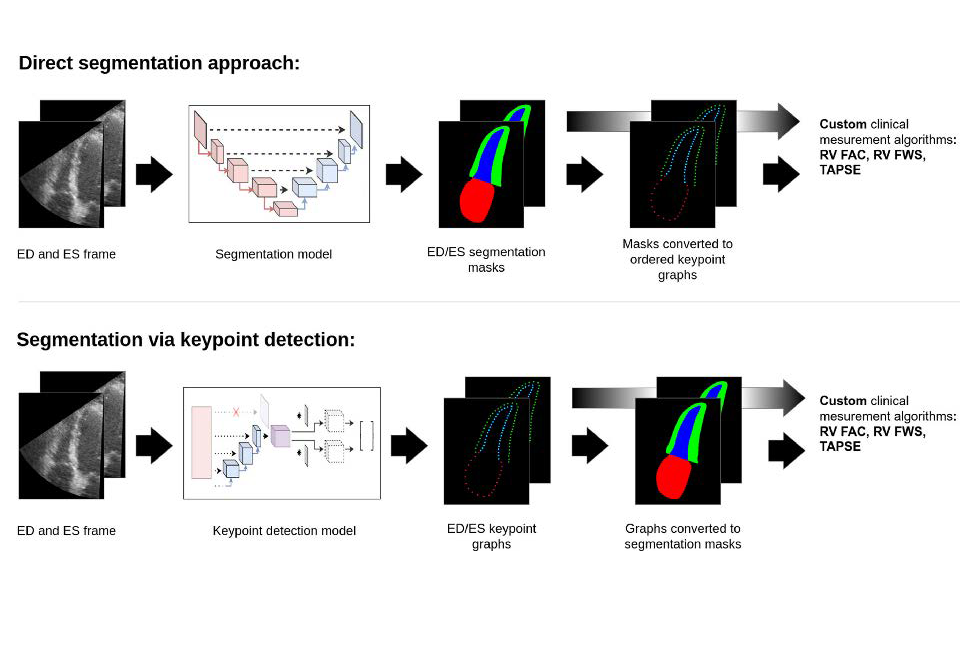
Illustrations of direct segmentation approach vs segmentation via keypoint detection
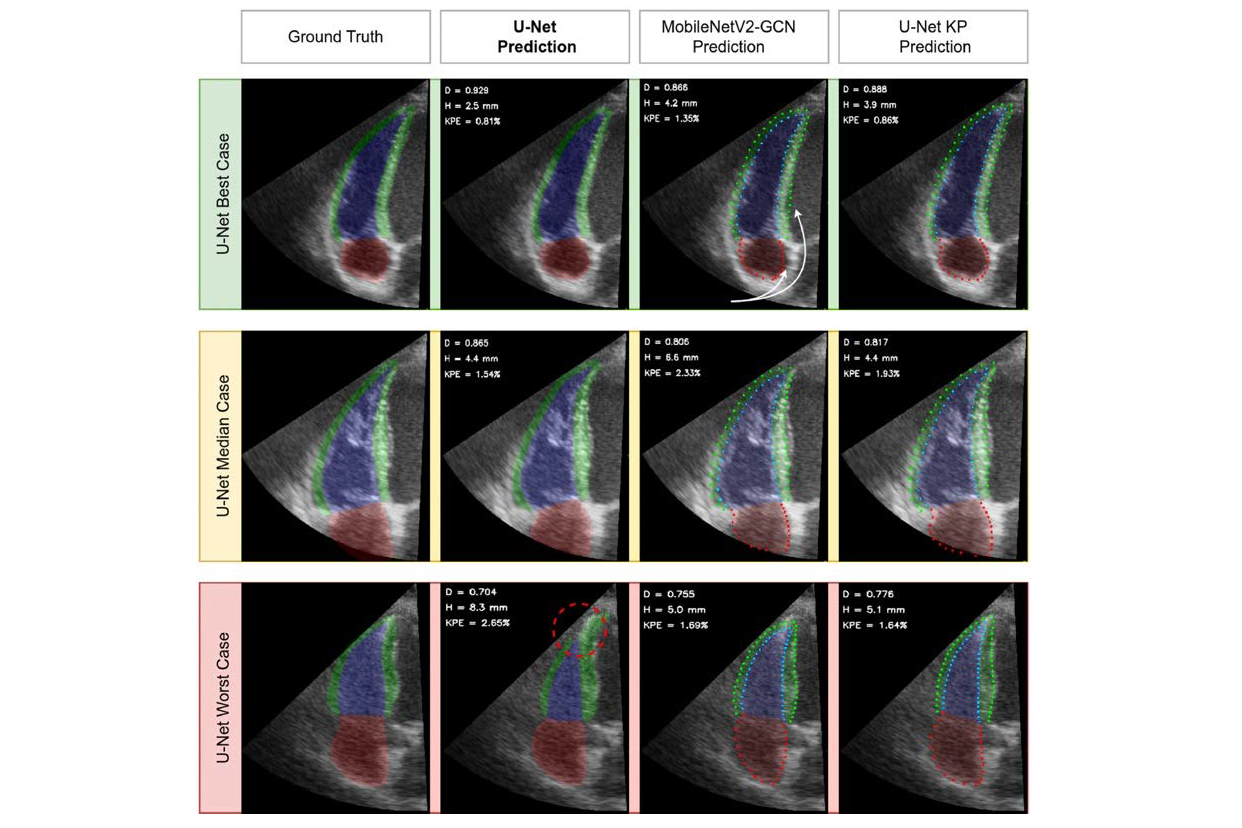
Illustrations of 2-D Echocardiography.
Activity completed, link:
https://doi.org/10.1016/j.ultrasmedbio.2023.12.018